Deep Learning Prediction of Adverse Drug Reactions in Drug Discovery Using Open TG–GATEs and FAERS Databases
Machine learning techniques are being increasingly used in the analysis of clinical and omics data. This increase is primarily due to the advancements in Artificial intelligence (AI) and the build-up of health-related big data. In this paper they have aimed at estimating the likelihood of adverse drug reactions or events (ADRs) in the course of drug discovery using various machine learning methods. They have also described a novel machine learning-based framework for predicting the likelihood of ADRs. Their framework combines two distinct datasets, drug-induced gene expression profiles from Open TG–GATEs (Toxicogenomics Project–Genomics Assisted Toxicity Evaluation Systems) and ADR occurrence information from FAERS (FDA [Food and Drug Administration] Adverse Events Reporting System) database, and can be applied to many ADRs. It incorporates data filtering and cleaning, as well as feature selection and hyperparameters fine-tuning. Using this framework with Deep Neural Networks (DNN), they built a total of 14 predictive models with a mean validation accuracy of 89.4%, indicating that their approach successfully and consistently predicted ADRs for a wide range of drugs. As case studies, they have investigated the performances of their prediction models in the context of Duodenal ulcer and Hepatitis fulminant, highlighting mechanistic insights into those ADRs. They have generated predictive models to help to assess the likelihood of ADRs in testing novel pharmaceutical compounds. They believe that their findings offer a promising approach for ADR prediction and will be useful for researchers in drug discovery.
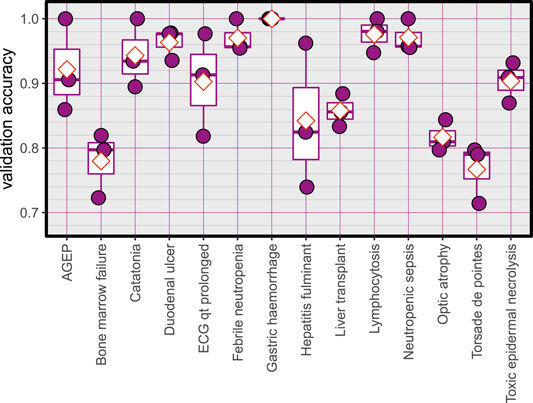
Validation accuracy of the created models, red diamonds represent the mean.
An adverse drug reaction (ADR) or event is defined as any unintended or undesired effect of a drug . ADR's are responsible for a high number of visits to emergency departments and in-hospital admissions. For instance, The Japan Adverse Drug Events (JADE) study reported around 17 adverse drug events per 1,000 patient days; 1.6% were fatal, 4.9% were life-threatening, and 33% were serious. These observations underscore the importance of toxicity assessment of any medication, especially in the early stages of drug discovery. Machine learning methods can play a significant role in the interpretation of various data types to predict ADRs. These methods utilize multiple kinds of input data, such as chemical structures, gene expressions as well as text mining. These data types are then processed algorithmically with random forest (machine learning) or by an artificial neural network (deep learning) to generate prediction models.
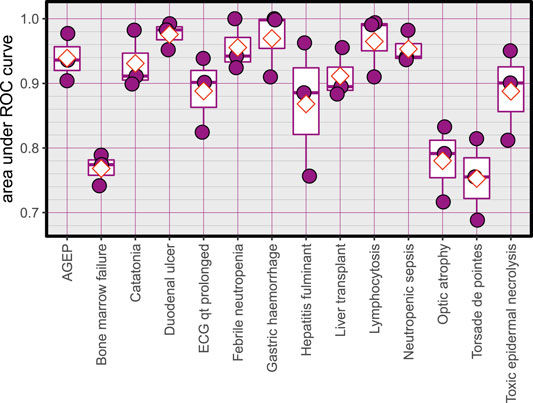
Area under ROC curves for the created models, red diamonds represent the mean.
Deep learning, a type of machine learning in Artificial intelligence (AI), has emerged as a promising and highly effective approach that can combine and interrogate diverse biological data types to generate new hypotheses. Deep learning is used extensively in the field of drug discovery and drug repurposing; however, its application in ADR prediction using gene expression data are rather limited. Open TG–GATEs is a large–scale toxicogenomics database that collects gene expression profiles of in vivo as well as in vitro samples that have been treated with various drugs. These expression profiles are an outcome of the Japanese Toxicogenomics Project, which aimed to build an extensive database of drug toxicities for drug discovery. It also collects physiological, biochemical, and pathological measurements of the treated animals. Similar databases that aim to profile compound toxicities have also been developed.
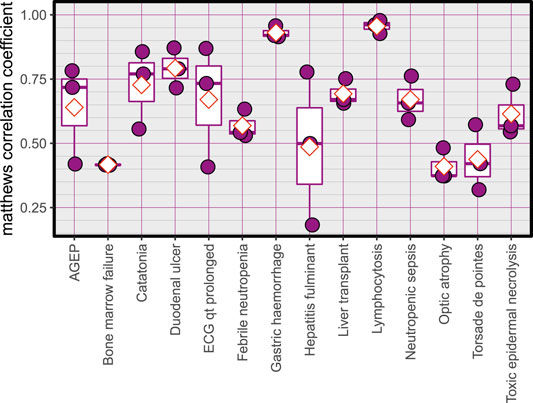
Matthews correlation coefficient, red diamonds represent the mean.
In contrast with other databases, such as (LINCS) , which have been used to predict multiple ADRs in a single study , Open TG–GATEs has been used to investigate individual/specific toxicities. To the best of their knowledge, no attempts have been made to provide a general framework for predicting multiple ADRs by using Open TG–GATEs.The design of Open TG-Gates has several advantages over the LINCS database, chiefly the inclusion of in vivo samples with different doses and durations of administration. Therefore, they designed their analysis to encompass multiple samples with different dosages and duration for each compound, necessitating additional noise-removal steps in the data processing. This study describes their approach to generating deep learning-based, systematic ADR prediction models. This approach combines ADR occurrence data, including frequency details, from the FAERS (FDA Adverse Event Reporting System) database, with the gene expression profiles from Open TG-GATEs. They show how to improve the models’ performance by applying feature selection and hyperparameter optimization algorithms. The methodologies and models described in their study offer valuable tools for assessing the likelihood of ADRs in the course of drug discovery.
Deep Learning Prediction of Adverse Drug Reactions in Drug Discovery Using Open TG–GATEs and FAERS Databases , Frontiers in Drug Discovery, 1, 2021. 10.3389/fddsv.2021.768792
Comments