Accurate Binding Configuration Prediction of a G-Protein-Coupled Receptor to Its Antagonist Using Multicanonical Molecular Dynamics-Based Dynamic Docking
They have performed dynamic docking between a prototypic G-protein-coupled receptor (GPCR) system, the β2-adrenergic receptor, and its antagonist, alprenolol, using one of the enhanced conformation sampling methods, multicanonical molecular dynamics (McMD), which does not rely on any prior knowledge for the definition of the reaction coordinate. Although they have previously applied their McMD-based dynamic docking protocol to various globular protein systems, its application to GPCR systems would be difficult because of their complicated design, which include a lipid bilayer, and because of the difficulty in sampling the configurational space of a binding site that exists deep inside the GPCR. Their simulations sampled a wide array of ligand-bound and ligand-unbound structures, and they measured 427 binding events during their 48 μs production run. Analysis of the ensemble revealed several stable and meta-stable structures, where the most stable structure at the global free energy minimum matches the experimental one. Additional canonical MD simulations were used for refinement and validation of the structures, revealing that most of the intermediates are sufficiently stable to trap the ligand in these intermediary states and furthermore validated their prediction results. Given the difficulty in reaching the orthosteric binding site, chemical optimization of the compound for the second ranking configuration, which binds near the pocket’s entrance, might lead to a high-affinity allosteric inhibitor. Accordingly, they show that the application of their methodology can be used to provide crucial insights for the rational design of drugs that target GPCRs.
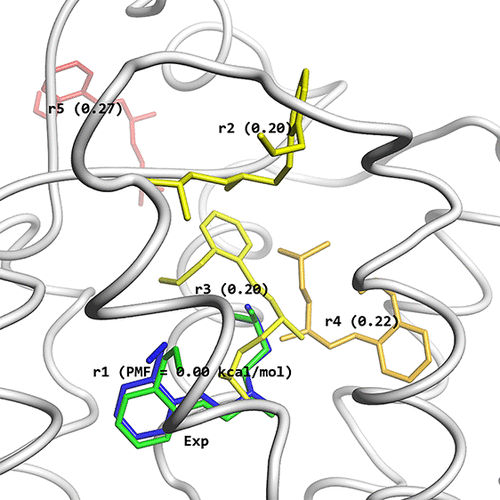
G protein-coupled receptors (GPCRs), which are triggered by their endogenous ligands, play crucial roles in sensory functions and many physiological processes, and thus they have long been considered to be potential drug targets. In the past decade, a substantial number of crystal structures of GPCRs have been solved, and the interactions between GPCRs, ligands, and G-proteins are also becoming clearer. However, GPCRs’ dynamic properties have only been studied to a limited degree, as it is not easy to capture them because of their complicated environment, considering that GPCRs are embedded within cell membranes.
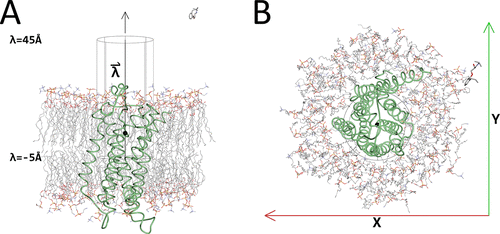
Initial McMD system with the cylinder. Shown are β2AR as a green tube model, with the membrane and the ligand alprenolol in CPK colors as stick models. Also shown is the cylinder with its axis that was used for restraining the ligand during the McMD-based dynamic docking simulations in black lines and the black sphere corresponds to the location of the ligand in the experimental structure, when projected onto the axis , which is parallel to the Z-axis. (A) Side view of the system. In addition, the positions of the lower limit (λ = −5 Å), the location of the experimental structure (λ = 5.27 Å) along the axis (black sphere), and the upper limit (λ = 45 Å) of the cylinder are also shown. (B) Top view of the system. Also shown are the X- and Y-axes. The image was produced using Molmil, a web-based molecular viewer developed by Protein Data Bank Japan.
Understanding the recognition mechanism, such as the ligand binding pathway and the free energy along this pathway that depend on the dynamic properties of the GPCRs, provides crucial information for the rational design of drugs, as it can help optimize the specificity of a ligand to the target binding site. While molecular dynamics (MD) simulations offer the possibility to investigate the binding mechanism by studying the entire conformational space, they cannot reach the timescales that biologically interesting phenomena occur at. Recently, docking using advanced MD has attracted much attention in the field of protein–drug interaction, where such methods have been coined dynamic docking. Enhanced conformational sampling MD methods such as multicanonical MD (McMD) accelerate the dynamics by applying a bias, enabling us to study phenomena such as the binding processes between molecules at atomistic resolution, not offered by either experimental methods or conventional MD simulations. With McMD, the bias (effectively the sampling temperature) is correlated to the potential energy, causing the McMD simulation to adaptively change the bias based on the density of states, where the unbiased canonical distribution can be reobtained via a reweighing scheme. Thus, the potential energy surface functions as a reaction coordinate, which does not depend on any prior knowledge, such as the bound complex structure, and can thus be used for a wide conformational search including binding configuration prediction. After obtaining the bias for McMD via preruns, a production run is executed in a wide temperature range to sample various configurations including bound ones. The canonical ensemble at any given temperature, which is one of the physico-chemically acceptable ensembles, can then be generated using a reweighting procedure. The free energy landscape (FEL), which governs the thermodynamic property of the system, can be obtained by mapping the structural ensemble onto a reaction coordinate such as a binding path or onto one or more principal components obtained by principal component analysis (PCA).
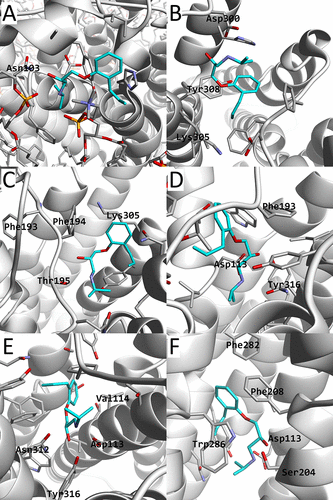
β2AR is shown as a white cartoon model, alprenolol, and its nearby residues shown as stick models. The carbon atoms of alprenolol are colored cyan. Also, several nearby residues are labeled. In (A), the membrane molecules (POPE, POPC, and cholesterol) are also shown. The images correspond to a structure picked in the regions of (X,Y) = (44,29), (26,25), (26,24), (33,28), (33,26), (30,26) in Å
Once the FEL has been assigned, not only the stable structures but also meta-stable ones along their formation paths can potentially be obtained from the trajectories.They have previously applied McMD to the conformational sampling of proteins and peptides, loop structure prediction of an antibody, and for the docking simulations between receptors and their ligands. Their McMD-based dynamic docking studies restrained the ligand inside a cylindrical region covering the binding site and the nearby bulk region and successfully generated the binding/unbinding path as well as the near-native binding configuration. They also succeeded in the accurate prediction of the binding affinity using path sampling methods, thermodynamic integration or umbrella sampling, along the path obtained from their McMD simulations.
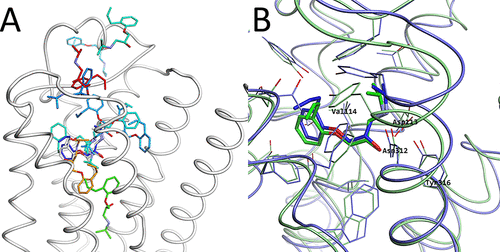
Structures obtained by the McMD-based dynamic docking simulations. (A) β2AR is shown as a white cartoon model and the ligand positions of rk are shown as stick models, colored based on their CFE in a blue-red gradient, where blue corresponds to a low CFE and red to a high one. (B) Comparison between the most meta-stable structure r1 (blue) predicted by the McMD-based dynamic docking simulations with the experimental structure (green). The residues that interact with the ligand are also shown, with some of them labeled.
Recently, they used dynamic docking simulations without cylindrical restraints, that is, where the ligand is allowed to exhaustively sample non-native binding sites, to demonstrate that they could not only accurately predict the binding site, but also the native binding configuration in such computationally complex cases. In this study, they have applied McMD-based dynamic docking simulations to study the structure and dynamics of a GPCR system, consisting of the β2-adrenergic receptor (β2AR) and its antagonist, alprenolol, in a lipid bilayer and with explicit solvent. Their system is similar to the pioneering work of Dror et al., who executed several very long unbiased MD simulations, although with many ligands per system, using Anton.Conversely, their dynamic docking simulation is based on biased sampling with only a single ligand to exhaustively explore the bound/unbound configurations. Starting from the unbound state, they successfully predicted the native bound configuration at the highest-ranking structure and in addition identified various meta-stable intermediary structures. This work shows that their methodology is also applicable to membrane-embedded proteins and can be used to provide crucial insight for the rational design of drugs that recognize GPCRs.
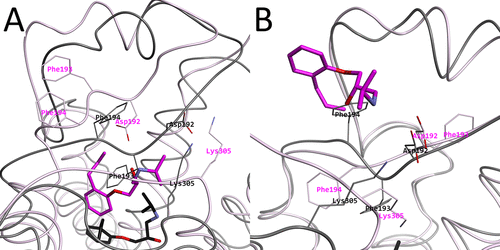
Intermediary structures near the high free energy regions. (A) Representative structure r264 picked from the 2D-FEL at (PC1, PC2) = (−50, −60) in magenta, with the experimental structure in black. (B) Representative structure r261 picked from the 2D-FEL at (PC1, PC2) = (100, −60) magenta, with the experimental structure in black. In both subfigures, Asp192, Phe193, Phe194, and Lys305 are shown and indicated as thin stick models.
Accurate Binding Configuration Prediction of a G-Protein-Coupled Receptor to Its Antagonist Using Multicanonical Molecular Dynamics-Based Dynamic Docking Gert-Jan Bekker, Mitsugu Araki, Kanji Oshima, Yasushi Okuno, and Narutoshi Kamiya Journal of Chemical Information and Modeling 2021 61 (10), 5161-5171 DOI: 10.1021/acs.jcim.1c00712
Comments